"When the flood is coming or leaving, how do people and the city adapt, react, improve the environment, and reflect on the transport network?"
The Metro Coastline project which aimed to tackle the challenges arising from rising sea levels and flooding that could affect the traffic system. Data science played a critical role in this project, which focused on the division of the metro line in 2050. The three main processes involved in this module were:
1. Background Learning: Collected data on existing bus information, history and weather data through API requests, which helped us understand how the sea level rise impacted people's accessibility to their destinations.
2. Real-time Computing: Utilized data science to provide timely updates on available bus stops and maps that reflected the sea level rise. The transit application showed changing routes and available stops in real-time while taking into account weather data inputs such as flooding, severe weather, or traffic jams.
3. Collaboration and Collection: We utilized APIs such as The Weather Channel to construct more stops based on factors like peak and off-peak time, population, and other data. Constructed new stops before the flooding occurred in appropriate locations, calculated using data. Used APIs to request data from weather prediction and Apple Maps to understand traffic and weather conditions in real-time. Worked on enhancing the flexibility of routes in the district with a changeable schedule based on activities, traffic conditions.
By leveraging data science, we created a sustainable and efficient transportation system that could adapt to changing environmental conditions while providing a better commuting experience.
#Unity #Csharp #DataScience #Infrastracture #APIcallout #Weatherstack #Dataset #NASA #Flooding #Application #UCLA #IDEO
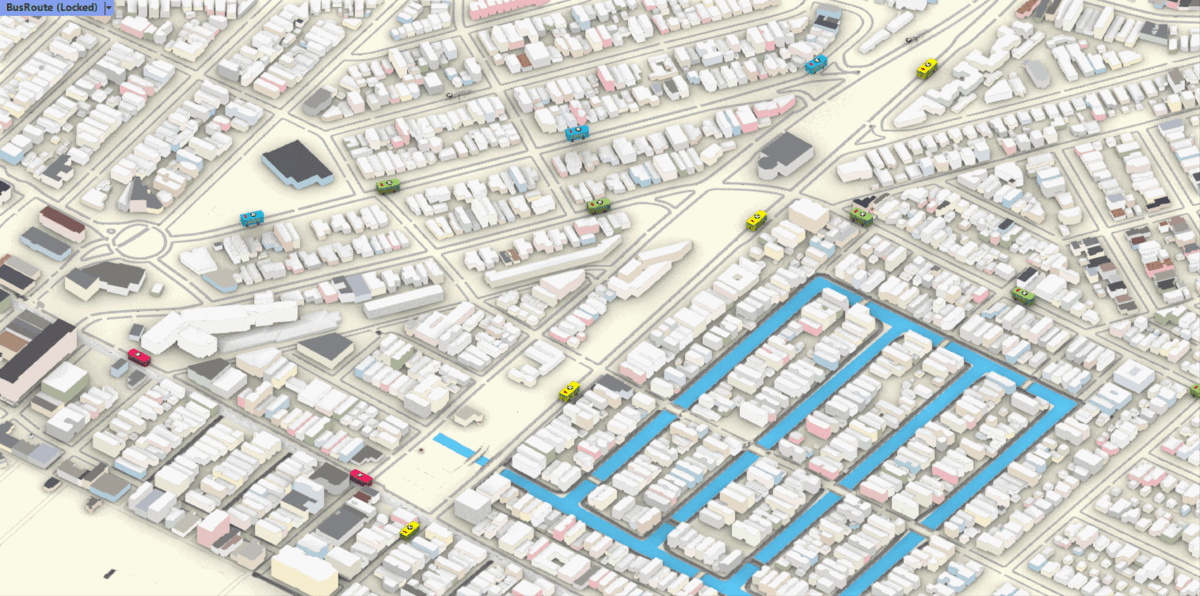